Selected Technical Expertise
In my work, I aim to develop simple and easily implementable solutions to complex big-picture problems that have broad implications for societal issues in the areas of renewable energy, medical diagnostics, electronic devices, and manned space exploration. This is achieved by combining intuition from experimental measurements with mathematical models and stochastic simulations. My current work is focused on nano-materials, specifically hybrid materials comprised of carbon nanotubes (CNTs) and graphene oxide (GO), and my technical expertise is strongest in three major areas that are further explained below: mathematical modeling and stochastic simulation of complex systems; predictive analytics for stronger and lighter advanced materials; process innovation for cheaper and faster material manufacturing.
Disclaimers: PDF documents provided on this webpage are for educational and personal use only. The work presented here was done in full during my tenure at Massachusetts Institute of Technology.
Quick links:
Mathematical Modeling | Predictive Analytics | Process Innovation | Funding Sources
Mathematical Modeling and Stochastic Simulation of Complex Systems
Many pivotal innovations in nanotechnology, such as faster more energy efficient processors for mobile computing, require design optimization and convergence that would be impossible to achieve within short time constraints using only empirical approaches, most notably trial and error. This is where the development and subsequent refinement of mathematical models and numerical simulations becomes imperative, since these tools can fast-track the iteration process by ensuring that expensive and time consuming experimentation is done in a highly targeted way. Additionally, these modeling and simulation tools can help assess the quality of the collected data in real-time, and ensure that any required alterations to the experimental design can be applied as they are discovered.
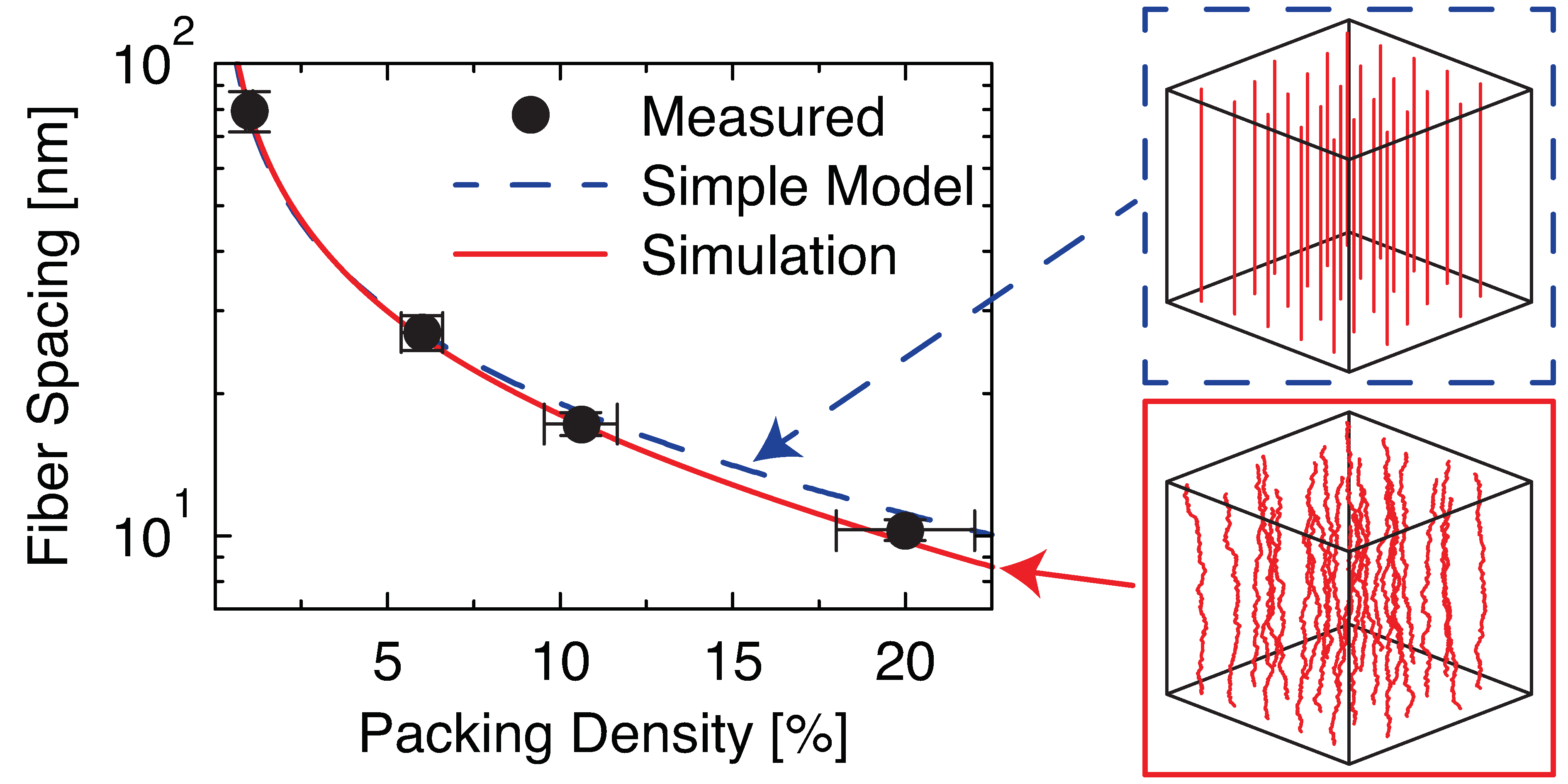
By developing a simple mathematical model, and subsequently a more representative stochastic numerical simulation, I was able to explain and predict how a complex property (the fiber spacing) of the nano-material I worked with evolves as its shape is being transformed as its packing density increases, which is a process that typically occurs during manufacturing of aerospace composite materials. See Figure 1 for a graphic summarizing the key finding from my PhD, and comparing the results of the simple mathematical model to those of the stochastic numerical simulation. As Figure 1 illustrates, for the few applications that require predictions with really high precision (< 1% error), the very accurate but computationally expensive stochastic numerical simulation is best, whereas for the vast majority of applications where ~ 5% - 10% error is acceptable, the simple but less representative mathematical model yields sufficiently precise predictions. Computationally guided materials design tools similar to the ones highlighted by Figure 1 are widespread throughout my work, and additional examples can be found in the relevant publications denoted below.
Related Press Releases:
- • nanotechweb.org: Impedance spectroscopy characterizes 1D nanostructures non-destructively.
- • nanotechweb.org: Stochastic CNT waviness shows nanocomposites can become stiffer.
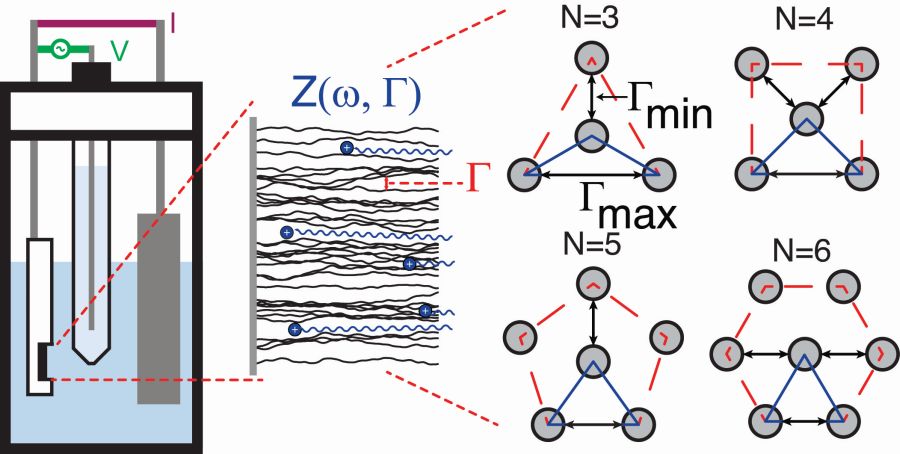
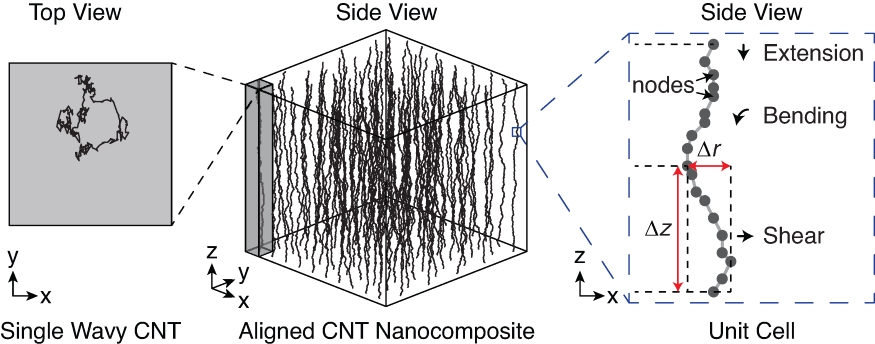
Relevant Publications:
- • H. K. Mutha, Y. Lu, I. Y. Stein, H. J. Cho, M. E. Suss, T. Laoui, C. V. Thompson, B. L. Wardle, and E. N. Wang, Porosimetry and packing morphology of vertically-aligned carbon nanotube arrays via impedance spectroscopy, Nanotechnology 28, 05LT01 (2017). | Download PDF | Supporting Information
- • I. Y. Stein, and B. L. Wardle, Mechanics of aligned carbon nanotube polymer matrix nanocomposites simulated via stochastic three-dimensional morphology, Nanotechnology 27, 035701 (2016). | Download PDF
- • I. Y. Stein, and B. L. Wardle, Packing morphology of wavy nanofiber arrays, Phys. Chem. Chem. Phys. 18, 694 (2016). | Download PDF | Supporting Information
- • I. Y. Stein, D. J. Lewis, and B. L. Wardle, Aligned carbon nanotube array stiffness from stochastic three-dimensional morphology, Nanoscale 7, 19426 (2015). | Download PDF | Supporting Information
- • I. Y. Stein, N. Lachman, M. E. Devoe, and B. L. Wardle, Exohedral Physisorption of Ambient Moisture Scales Non-monotonically with Fiber Proximity in Aligned Carbon Nanotube Arrays, ACS Nano 8, 4591 (2014). | Download PDF | Supporting Information
- • I. Y. Stein, and B. L. Wardle, Morphology and processing of aligned carbon nanotube carbon matrix nanocomposites, Carbon 68, 807 (2014). | Download PDF | Supporting Information
- • H. Cebeci*, I. Y. Stein*, and B. L. Wardle, Effect of nanofiber proximity on the mechanical behavior of high volume fraction aligned carbon nanotube arrays, Appl. Phys. Lett. 104, 023117 (2014). | Download PDF | Supporting Information
- • I. Y. Stein, and B. L. Wardle, Coordination number model to quantify packing morphology of aligned nanowire arrays, Phys. Chem. Chem. Phys. 15, 4033 (2013). | Download PDF | Supporting Information
Predictive Analytics for Stronger and Lighter Advanced Materials
The most significant challenge that hinders the use of new technology, especially nanotechnology based solutions, is that without a proven track record of a technology's lifespan and long-term failure characteristics, there is significant risk and potential safety and/or compliance issues associated with its adoption. A common approach for corporations to address such an uncertainty and minimize the associated risk is to expose a new advanced material to grueling long-term testing lasting a decade or more. A prime example of such a process took place in the Aerospace industry, where carbon fiber based composite materials took several decades to go from the drawing board to comprising 50% or more of the commercial passenger aircraft's structure (e.g., the recently released Boeing 787 Dreamliner and Airbus A350 XWB). While such extended testing is commonly required to meet existing safety regulations, without sophisticated predictive analytics, there could be a large number of failure mechanisms that were never tested, and remain undiscovered until the product enters the marketplace. For example, in the Health and Life Science industry, this could lead to a pharmaceutical drug being pulled from shelves after a severe and previously unknown side effect is discovered by early users.
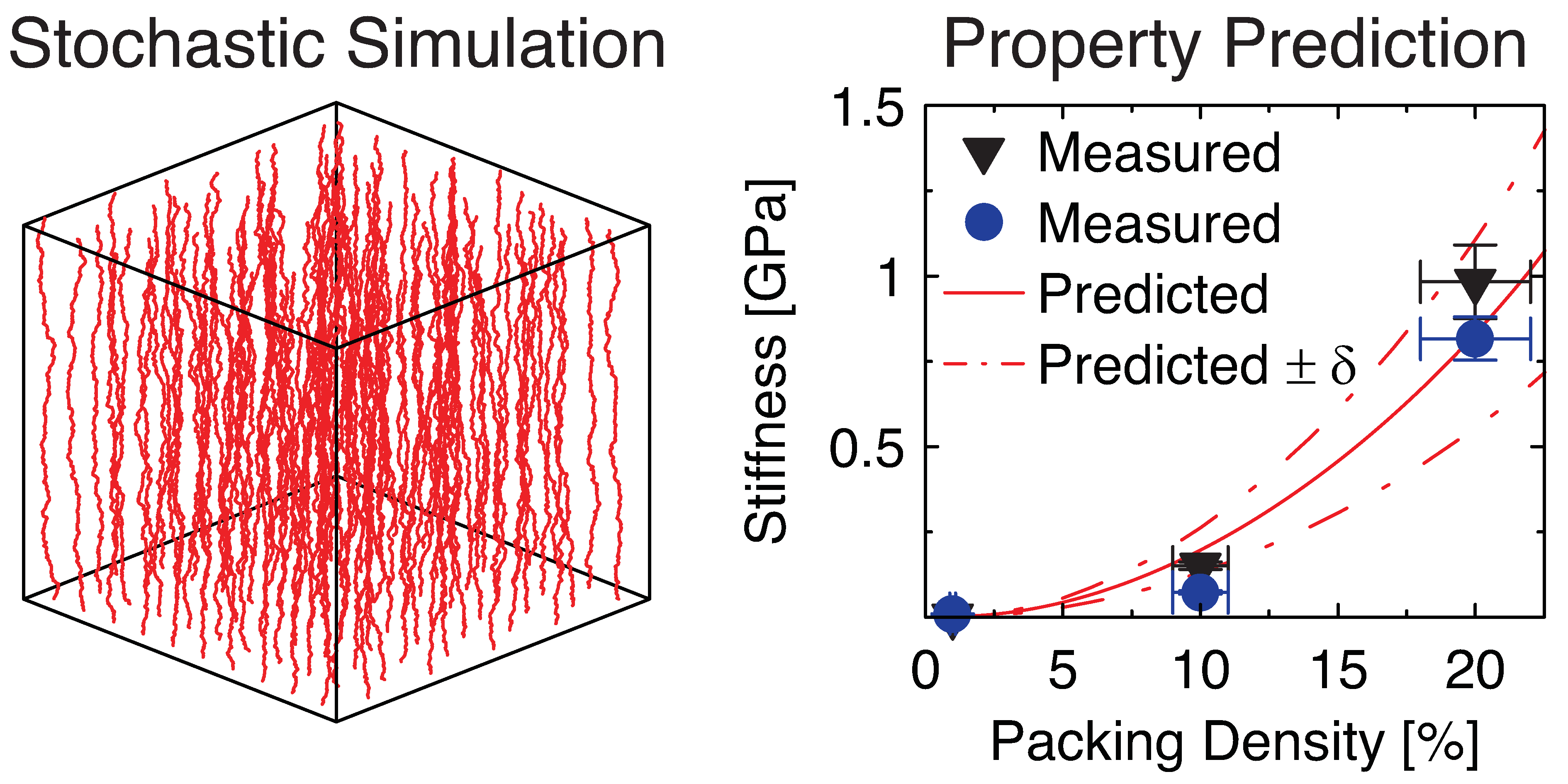
By reducing complex big-picture problems into multiple parts that could be individually addressed using mathematical models and stochastic numerical simulation, I was able to quantify and predict the behavior of the nano-material that I worked with as it was being exposed to external forces, which is highly relevant to how this material would react if used within components of commercial aircraft. See Figure 2 for a graphic summarizing key property prediction findings from my PhD, which shows that by modeling and simulating the individual parts that contribute to the stiffness of nano-materials, their behavior when exposed to external forces can be effectively predicted. As Figure 2 illustrates, since representative property predictions tools can account for the key factors whose combination yields the observed behavior, such tools could be invaluable to exploring conditions that might be difficult or extremely time consuming to explore experimentally, but might rarely occur in practice, to test for any unlikely but possible failure mechanisms that would be overlooked otherwise.
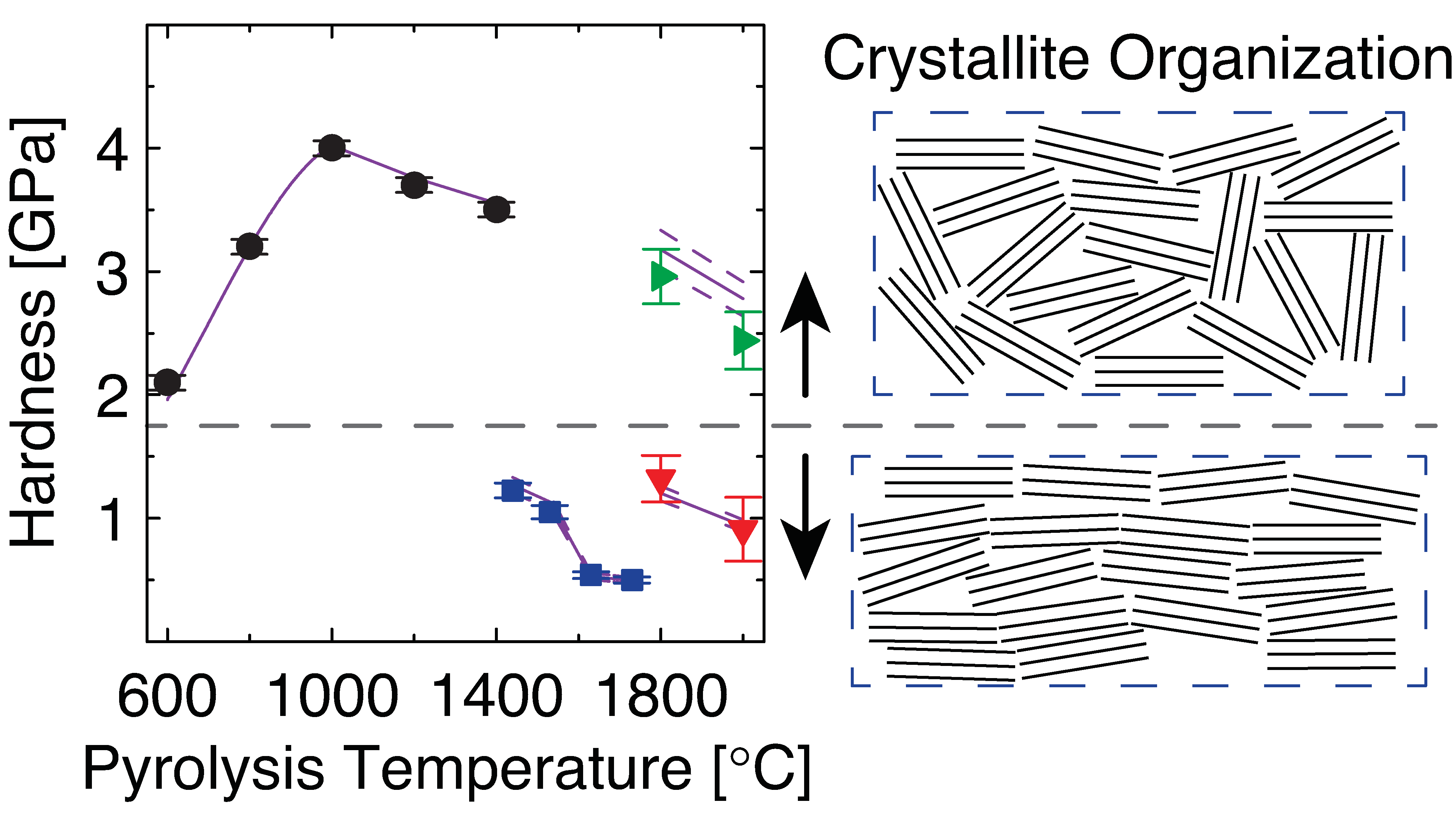
Also, by connecting the strength of disordered carbon materials with the temperature at which they were manufactured, I discovered that processing at higher temperatures, which can become very expensive, does not necessarily lead to enhanced performance. See Figure 3 for a graphic outlining key findings from my PhD that tie disorder and processing temperature to a technical measure of strength known as hardness, which shows that there exists an optimum temperature at ~ 1000 degrees Celsius above which strength is diminished. As Figure 3 demonstrates, since property prediction tools could be used to refine manufacturing conditions without compromising material performance, they enable process optimization mediated cost-savings that facilitate the use of advanced materials in industrial applications. Property prediction tools similar to the one highlighted by Figures 2 and 3 are present throughout my work, and additional examples can be found in the relevant publications denoted below.
Related Press Releases:
- • MIT News: Disorder can be good.
- • nanotechweb.org: Stochastic CNT waviness shows nanocomposites can become stiffer.
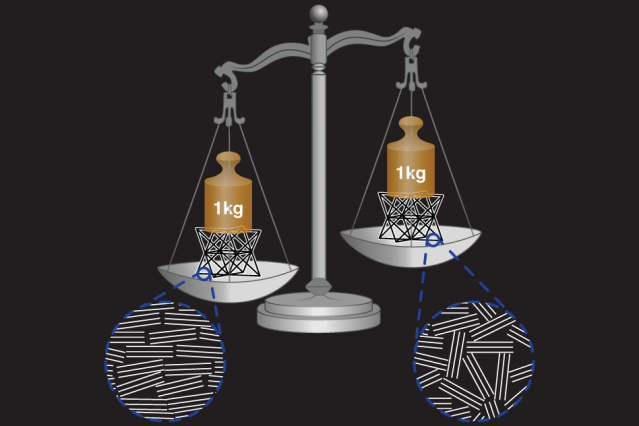
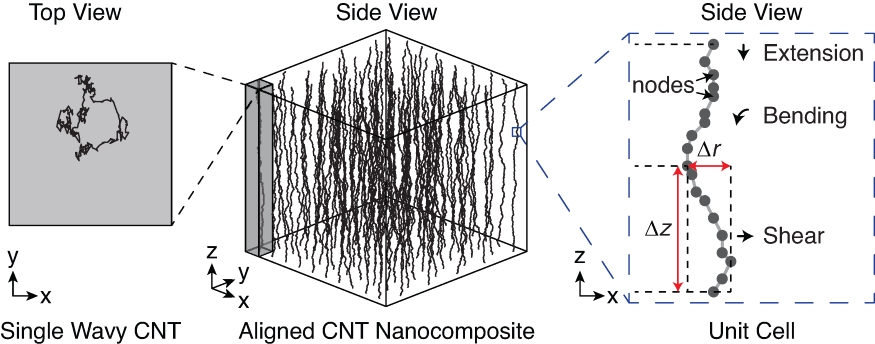
Relevant Publications:
- • I. Y. Stein, A. J. Constable, N. Morales-Medina, C. V. Sackier, M. E. Devoe, H. M. Vincent, and B. L. Wardle, Structure-mechanical property relations of non-graphitizing pyrolytic carbon synthesized at low temperatures, Carbon 117, 411 (2017). | Download PDF | Supporting Information
- • I. Y. Stein, and B. L. Wardle, Mechanics of aligned carbon nanotube polymer matrix nanocomposites simulated via stochastic three-dimensional morphology, Nanotechnology 27, 035701 (2016). | Download PDF
- • I. Y. Stein, D. J. Lewis, and B. L. Wardle, Aligned carbon nanotube array stiffness from stochastic three-dimensional morphology, Nanoscale 7, 19426 (2015). | Download PDF | Supporting Information
- • H. Cebeci*, I. Y. Stein*, and B. L. Wardle, Effect of nanofiber proximity on the mechanical behavior of high volume fraction aligned carbon nanotube arrays, Appl. Phys. Lett. 104, 023117 (2014). | Download PDF | Supporting Information
- • D. Handlin*, I. Y. Stein*, R. Guzman de Villoria, H. Cebeci, E. M. Parsons, S. Socrate, S. Scotti, and B. L. Wardle, Three-dimensional elastic constitutive relations of aligned carbon nanotube architectures, J. Appl. Phys. 114, 224310 (2013). | Download PDF
Process Innovation for Cheaper and Faster Material Manufacturing
Although possessing the vision for a better approach to solve a complex societal problem is essential, such insight is of little practical use if the solution cannot be implemented in a consistent, cost-effective, and scalable way. If a sufficient number of these conditions is not met, many companies typically invest heavily in internal taskforces and/or external consultants with the objective of overcoming these challenges via major process innovations. However, as discussed previously, if these teams do not have access to sufficiently advanced predictive analytics and data processing automation tools, their effectiveness could be significantly diminished within the time and financial constraints set by the company's leadership. This is where being able to effectively break-down the big-picture problem into its key individual components is essential. Since many smaller component problems could be addressed by either adapting existing solutions to related problems or by making reasonable assumptions to be revisited when more data is available, a team whose leadership can fluently translate a large challenge into small actionable tasks is best equipped to tackle complex and pivotal multi-part problems.
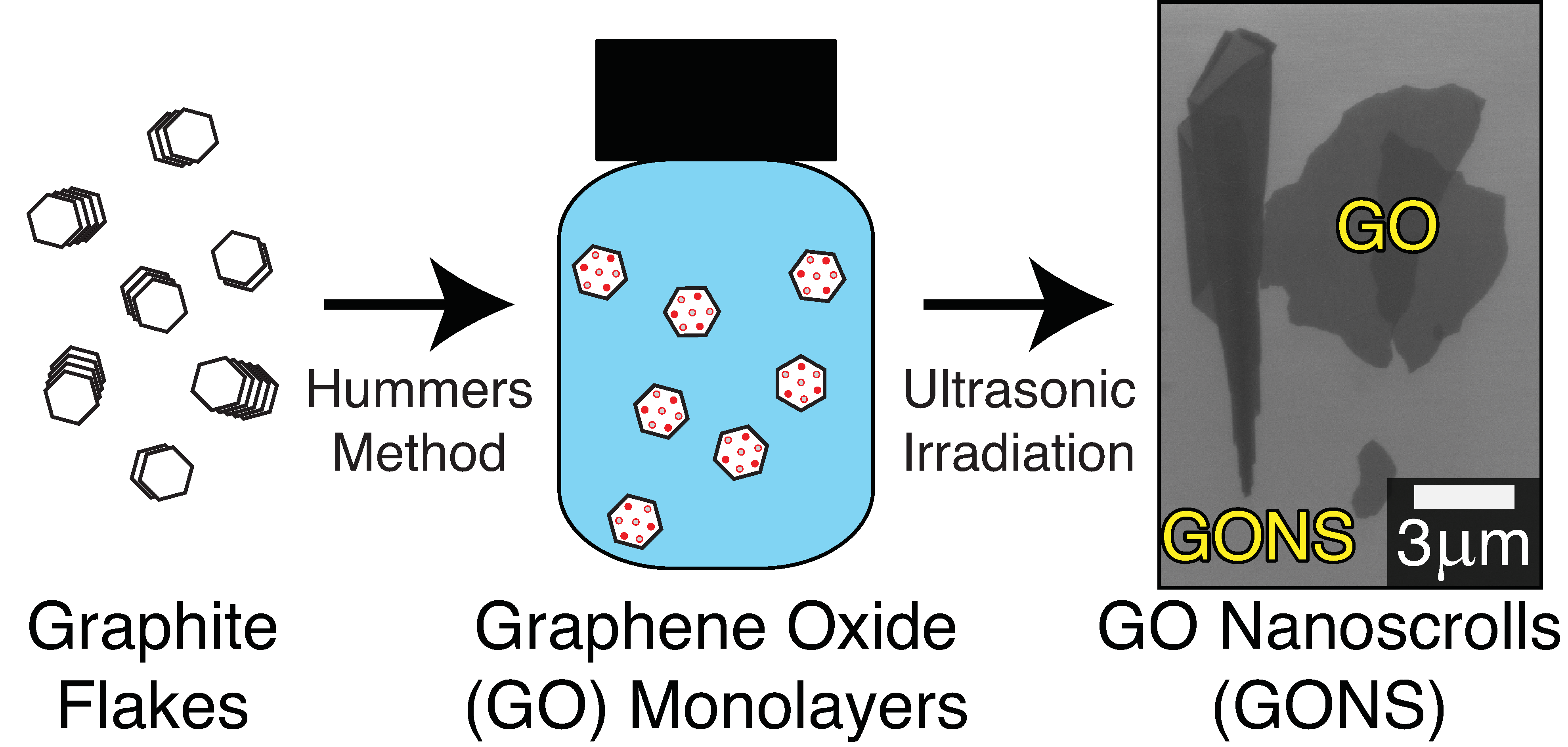
By co-leading a team comprised of researchers from Harvard University and MIT, my inter-disciplinary team was able to precisely modify a process that commonly used to prepare nano-materials for water filtration membranes and electronic devices so that their geometry was transformed from a flat film into a scroll-like hierarchy. See Figure 4 for a graphic summarizing a process innovation that can turn graphite 'flakes' into nano-scale 'scrolls'. Since we were able to effectively pinpoint the most important factors, irradiation time and power, that govern the transformation of these nano-scale films into 'scrolls', and since mathematical models were developed to better quantify and predict how different power level and exposure times would impact the nano-materials, this report contributes valuable insight that can enable such nano-scale 'scrolls' to be manufactured cheaply and in large quantities by commercial entities.
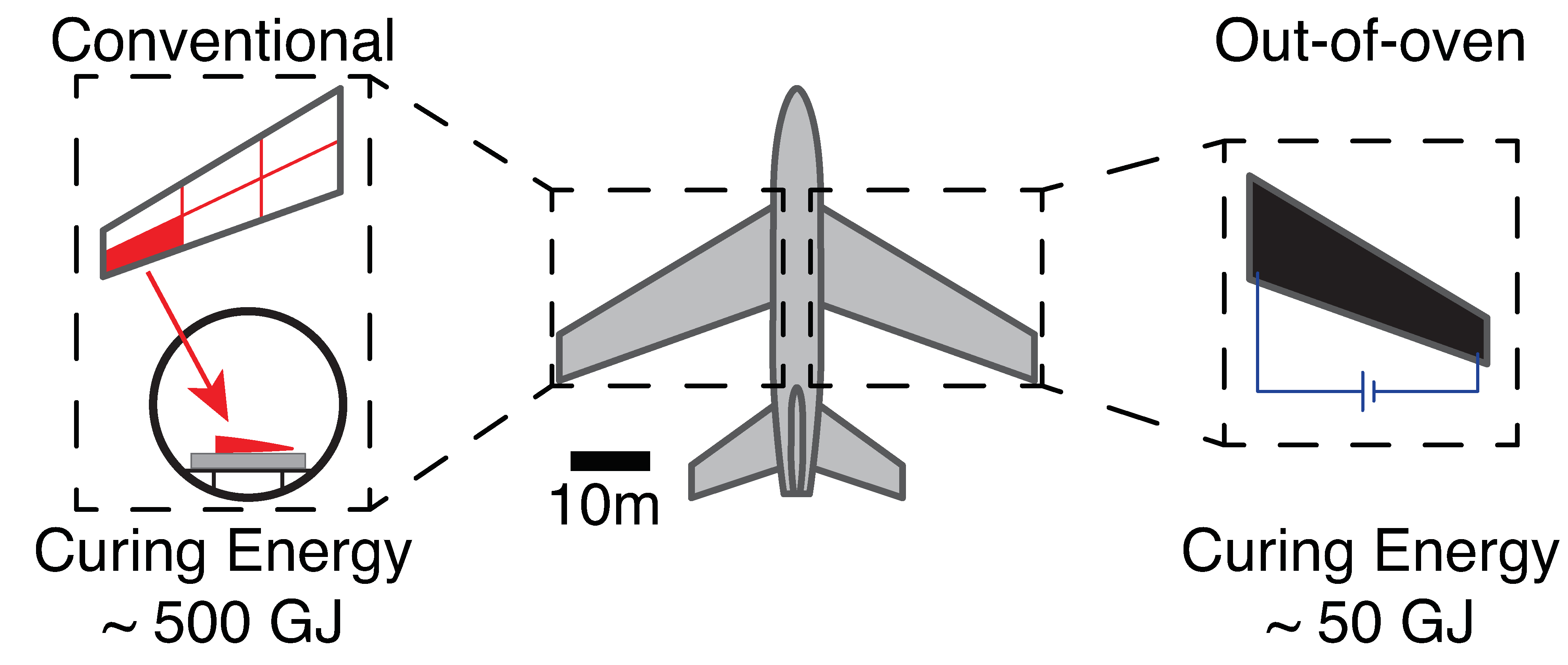
Additionally, by co-leading a team of researchers from MIT and industry, my team was able to develop a new approach for manufacturing aerospace composite materials without using a large and energy inefficient 'autoclave' oven, and thereby saving more than 90% of the energy required for making state-of-the-art aircraft components. See Figure 5 for a graphic comparing the conventional oven-based manufacturing approach to the new and more energy efficient 'out-of-oven' manufacturing approach. Since the energy required for heating the composite material parts during manufacturing accounts for ~ 25% - 50% of their total acquisition cost, by identifying and subsequently reducing the energy consumed during the heating steps by >90%, this process innovation can lead to signifcantly reduced cost of building commercial airplanes. Process innovations similar to the ones highlighted by Figures 4 and 5 are integral to other aspects of my, and additional examples can be found in the relevant publications denoted below.
Related Press Releases:
- • MIT News (Featured on MIT Homepage): For stronger, lighter, cheaper materials, scroll up.
- • MIT News: Taking aircraft manufacturing out of the oven.
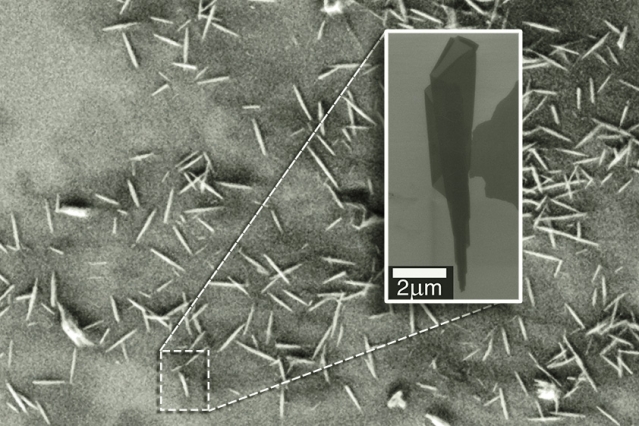
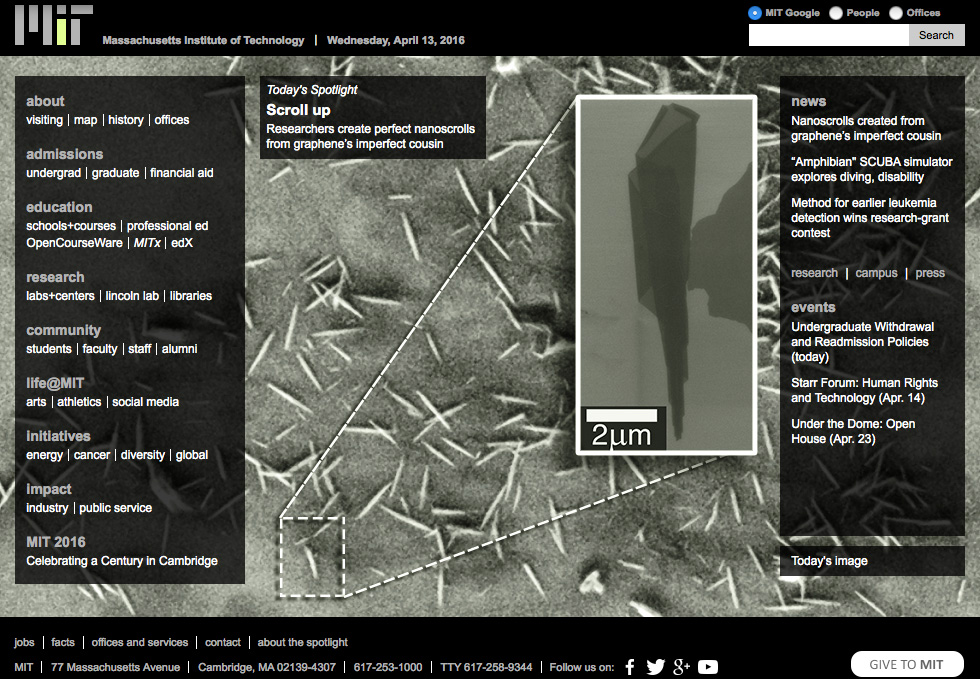
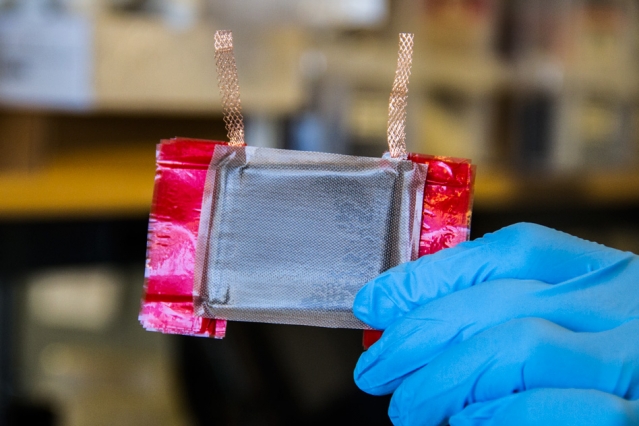
Relevant Publications:
- • C. A. Amadei*, I. Y. Stein*, G. J. Silverberg, B. L. Wardle, and C. D. Vecitis, Fabrication and morphology tuning of graphene oxide nanoscrolls, Nanoscale 8, 6783 (2016). | Download PDF | Supporting Information
- • I. Y. Stein, D. J. Lewis, and B. L. Wardle, Aligned carbon nanotube array stiffness from stochastic three-dimensional morphology, Nanoscale 7, 19426 (2015). | Download PDF | Supporting Information
- • J. Lee*, I. Y. Stein*, S. S. Kessler, and B. L. Wardle, Aligned Carbon Nanotube Film Enables Thermally Induced State Transformations in Layered Polymeric Materials, ACS Appl. Mater. Interfaces 7, 8900 (2015). | Download PDF
- • J. Lee*, I. Y. Stein*, M. E. Devoe, D. J. Lewis, N. Lachman, S. S. Kessler, S. T. Buschhorn, and B. L. Wardle, Impact of carbon nanotube length on electron transport in aligned carbon nanotube networks, Appl. Phys. Lett. 106, 053110 (2015). | Download PDF | Supporting Information
- • I. Y. Stein, N. Lachman, M. E. Devoe, and B. L. Wardle, Exohedral Physisorption of Ambient Moisture Scales Non-monotonically with Fiber Proximity in Aligned Carbon Nanotube Arrays, ACS Nano 8, 4591 (2014). | Download PDF | Supporting Information
- • I. Y. Stein, and B. L. Wardle, Morphology and processing of aligned carbon nanotube carbon matrix nanocomposites, Carbon 68, 807 (2014). | Download PDF | Supporting Information
Funding and Support
This work was made possible through the generous financial support provided by the following organizations:
- • Department of Defense (DoD) through the National Defense Science & Engineering Graduate (NDSEG) Fellowship Program.
- • National Science Foundation (NSF).
- • U.S. Army Research Office (ARO).
- • Nano-Engineered Composite aerospace STructures (NECST) Consortium at MIT.